The Truth About AI
True, AI can significantly boost performance and revenues by transforming organisations in lots of different ways. From how they engage with customers to how they recruit people and manage their finances. In fact, it’s predicted to boost GDP in the UK by 14% come 2030.
But honestly speaking, AI is anything but plug-and-play. And maybe that is why we see an astonishing 85% of AI projects fail to deliver the expected business value.
Something doesn’t add up. You seemingly have the data and access to the AI models, so what’s wrong? Well, let’s go back to the data for a second—because that’s where AI projects normally go off the rails.
Don’t forget the data
Broadly speaking, either companies don’t have enough of it, aren’t using it in the right way, have major quality issues, or just don’t have the correct systems to store and warehouse the stuff. We see the same problems time and again.
When it comes to artificial intelligence, getting the foundations right is absolutely critical. AI isn’t a quick process and there isn’t a ‘one size fits all’ solution; this is a long-term strategic investment in your business which will improve over time. However, all this relies on the quality of your model inputs, namely data. If you’re not getting this right, you’re already setting yourself up for failure (and a lot of wasted time, effort and money).
In this blog we’re going to tell you how to prepare your data for AI success. With our 12 steps, you will be able to navigate AI with confidence and start reaping the full power of the technology for better business results.
Here we go:
#1 Data volumes
Generally, AI algorithms require significant volumes of data – we really can’t emphasise this enough. However, just how much will depend on the AI use case you’re focused on. One figure often referred to is the need for 10x as many rows (data points) as there are features (columns) in your data set. The baseline for the majority of successful AI projects is normally more than 1 million records for training purposes.
#2 Data history
Let’s say you want to use AI for demand forecasting or for marketing mix models. In this case, at Ipsos Jarmany, we recommend having at least 3 years’ worth of data; otherwise, your model will just repeat the previous year’s outputs. It stands to reason that for AI to detect and predict events better than we can, it needs to work with loads of historical data to uncover the patterns and anomalies that we need it to.
#3 Data relevance
Depending on your use case, you’ll also need specific data sets for your algorithm. For example, marketing mix models aims to measure the impact of various marketing inputs on sales and market share, hence you’ll need data sets such as previous years’ sales, marketing performance and budget allocations.
#4 Data Quality
We’ve put this at #4 but maybe we should have put it at #1. It’s massive. If the quality of data you’re inputting into your AI model is poor, you can bet your chances that the AI models output will be poor.
In short, many companies face data quality issues, so there’s every chance your unsuccessful AI project will do nothing more than put a broader issue under the spotlight. Not a bad thing.
So, how do you go about achieving data quality? Essentially, you’re going to have to go through your data and ensure it doesn’t suffer from any of the following:
• Inconsistency
• Duplication
• Inaccuracy
• Outdatedness
• Irrelevancy
• Incompleteness
• Lack of governance
#5 Data Understanding
Whilst we place a massive emphasis on data quality (and rightly so), having a large volume of high-quality data doesn’t stand for much if you don’t have a solid understanding of your data. By this we mean understanding what the data relates to, what the data is telling you, and being able to identify patterns and trends, as well as spikes, dips and outliers in your performance.
Additionally, when it comes to data, it’s key that you have an understanding of what’s happening within the wider business so you can apply business context to the data. For example, if you’re seeing a dip in sales performance can this be attributed to seasonality, or perhaps a stock or distribution issue?
#6 Data labelling
This is pretty much as it sounds. You’re annotating your data, defining it as an image, text, video or audio, to help your learning model find “meaning” in the information. It’s important to remember that labelling—like the next step we’ll go on to talk about—should come after you’ve ensured the data quality.
Labelling is essentially a manual step done by software engineers and the last thing you want is for an engineer to waste their time labelling duplicated, inaccurate or irrelevant data.
#7 Data augmentation
Data augmentation is all about creating new or modified data from your existing sets to artificially increase the quantity of data and its value.
By making small changes such as randomly changing words in text data, you’re not only increasing the data set but improving its quality, helping avoid “overfitting”, where your model aligns too closely to your original training data and struggles with new information.
#8 Data systems
For AI to work, you’re going to need the right data systems in place. The key essentials include loads of computing capacity, offering a mix of CPU and GPU processing, modern storage and warehousing, high bandwidth, low latency networking and security for your sensitive data.
That’s potentially a lot of investment, and therefore many companies are looking at cloud services to give them the systems they need at the right price. Leading cloud providers, including Microsoft, can provide you with AI data systems you require to get your AI project off the ground.
#9 Data privacy
Data privacy is more tightly controlled than ever and rightly so. Yet, as we know, AI needs tons of data to work, which amplifies your risk of privacy breaches occurring. Trust us, you need to take data privacy very seriously and invest in the tools and techniques to make sure your data comes with encryption, anonymisation and owner consent.
#10 Data governance
We touched on this earlier when we talked about data quality. The point we made then was that the correct data governance will boost your data quality, saving you time and money. What’s more, correct governance will ensure sensitive and confidential data is classified accordingly and deleted in line with the appropriate data retention schedule.
#11 Data People
Another key step that you need to consider on your journey to becoming AI-ready is data people; and there are three sides to this point.
Firstly, gaining internal buy-in from the key stakeholders within your business is critical to any AI project. These stakeholders need to share the same vision as you when it comes to what you’re trying to achieve with AI and how it can benefit the business. They need to understand the strengths and limitations of the AI model so that expectations are aligned. And, the only way you can ensure internal adoption is by getting stakeholder buy-in from the get-go.
Secondly, in any digital transformation project roughly 10% is based on having the right tech in place, and 90% is based on having the right people and skillsets in place. This may seem surprising, given the importance of having the right tech stack to handle your data and AI models, however that said, you really can’t afford to underestimate how important it is to have the right people in place too. It’s certainly not new news that there’s a skills gap in the industry right now, so in order to future-proof your AI strategy, you need to consider what skill sets you currently have within the business, identify areas where training and development is required, and establish at what point you may need to lean on external agencies for support.
Lastly, is data culture. It’s true, a lot of people are concerned that their jobs will become obsolete as a result of businesses adopting AI. In fact, 44% of employees are worried about the impact on AI on their jobs. Given this, fostering a strong data culture within your organisation should be a priority if you want to ensure internal adoption of your AI model, and offset the workforce anxiety associated with AI. If your workforce are invested in your AI strategy, then this will set you off with a solid foundation for achieving AI success.
#12 Data automation
Now that we’re coming to the end, and you’re clear on what you need to do, we’re going to put the idea of automation on the table. It makes a lot of business sense to remove the human intervention here. To use an example, AI Builder, as part of Microsoft Power Platform, offers a turnkey solution for using Microsoft AI through a point-and-click experience. It’s being used by many large enterprises for hand off, error free AI models.
Data happiness
No doubt, that feels like a long list, and you’re right, it is. But as you’d expect there are tools out there to help businesses get their data in the right order for AI.
What’s more, at Ipsos Jarmany, we have the data engineering and AI expertise to help you apply those tools, flesh out your data strategy, and get your data AI-ready to start maximising business growth and efficiencies. If that weren’t enough, we have the AI skills to build the ML models that will extract all the value you need from your data.
Today, we’re helping many companies successfully integrate AI into their businesses, making certain their data is up to the job.
If you’d like to know more about data or AI please get in touch.
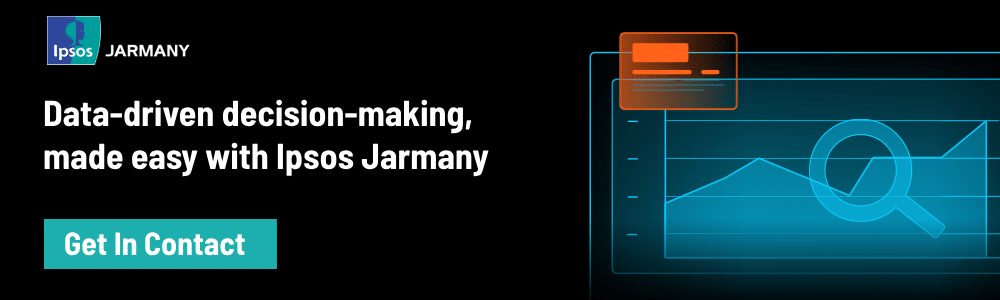